Retrieval-Augmented Generation (RAG) is a transformative technique that enhances the capabilities of artificial intelligence (AI) by integrating external knowledge sources. This innovative approach is poised to revolutionize how businesses leverage AI to gain a competitive edge.
RAG combines two core components: retrieval and generation. The retrieval component acts as a smart search engine, identifying relevant documents or passages from a vast knowledge repository based on a user’s query. The generation component, often an advanced language model, then uses this curated information to produce coherent and contextually appropriate responses.
By merging these elements, RAG overcomes the limitations of traditional language models, offering several notable advantages:
- Improved Accuracy: RAG can boost the precision of AI-generated outcomes by anchoring them in verified knowledge bases, reducing the likelihood of misinformation.
- Scalability: RAG systems can efficiently handle large datasets, making them suitable for various applications across different sectors.
- Flexibility: This technique can be applied to diverse tasks, including question answering, conversational AI, and document summarization, where context and up-to-date information are vital.
- Enhanced User Trust: By allowing AI models to reference authoritative sources, RAG increases user confidence in AI-generated outputs.
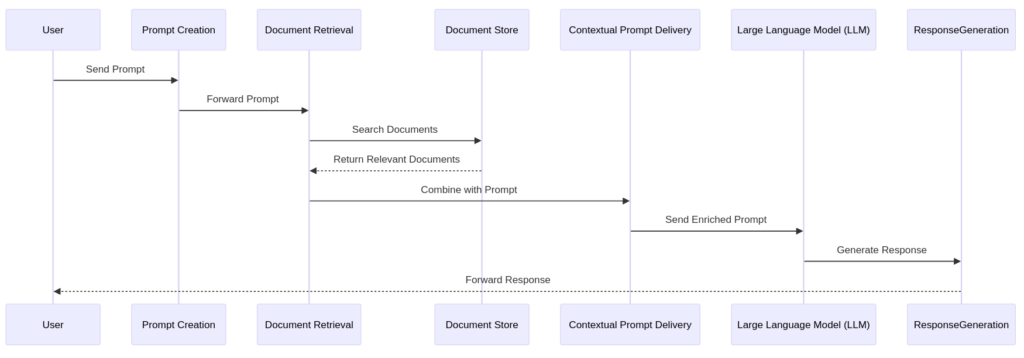
This diagram provides a clear visual representation of how the RAG architecture enhances the capabilities of LLMs by integrating real-time data retrieval into the response generation process.
Real-World Examples of RAG in Action
- Healthcare: In the healthcare sector, RAG models can support doctors in making informed decisions by retrieving up-to-date medical literature, patient histories, and treatment guidelines. By integrating relevant information from various sources, RAG can help healthcare professionals provide more accurate diagnoses and personalized treatment plans.
- Legal Services: Legal professionals can leverage RAG to access case law, statutes, and legal articles efficiently, streamlining their research processes and enhancing the accuracy of their arguments. For instance, a corporate law firm can use RAG to train AI on past precedents, enabling lawyers to quickly find relevant cases that support their arguments or providing instant answers during due diligence processes.
Challenges in Implementing RAG
While the benefits of RAG are clear, implementing this technology comes with its own set of challenges. CEOs and CTOs embarking on RAG implementation may face several hurdles:
- Data Privacy and Security: Ensuring the confidentiality of sensitive data is a critical concern when deploying RAG systems. Enterprises must adopt robust data protection measures and comply with relevant regulations.
- Scalability and Flexibility: Achieving scalable RAG with the meaningful flexibility inherent to the use case and business requires a well-defined strategy. Enterprises must carefully plan how to best scale AI with RAG to ensure long-term effectiveness.
- Cost and Resource Efficiency: While RAG systems are generally more cost-effective than training large models from scratch, the initial implementation costs can be significant. Enterprises must carefully evaluate the potential return on investment before committing resources.
Conclusion
As organizations increasingly recognize the importance of leveraging their knowledge reservoirs to gain a competitive edge, RAG is poised to become a pivotal element of data-driven decision-making. By amalgamating cloud deployment, edge frameworks, and state-of-the-art language models, RAG is unlocking new realms of AI-driven intelligence.
Whether enhancing healthcare outcomes, streamlining legal research, personalizing customer experiences, or empowering data-driven strategies, RAG is reshaping how businesses interact with and benefit from AI technology. As RAG matures, it holds the potential to bridge the gap between the vast internet knowledge and the unique expertise and data within organizations, paving the way for a future where artificial intelligence becomes an even more integral and transformative force in our lives.